Кракен актуальное зеркало
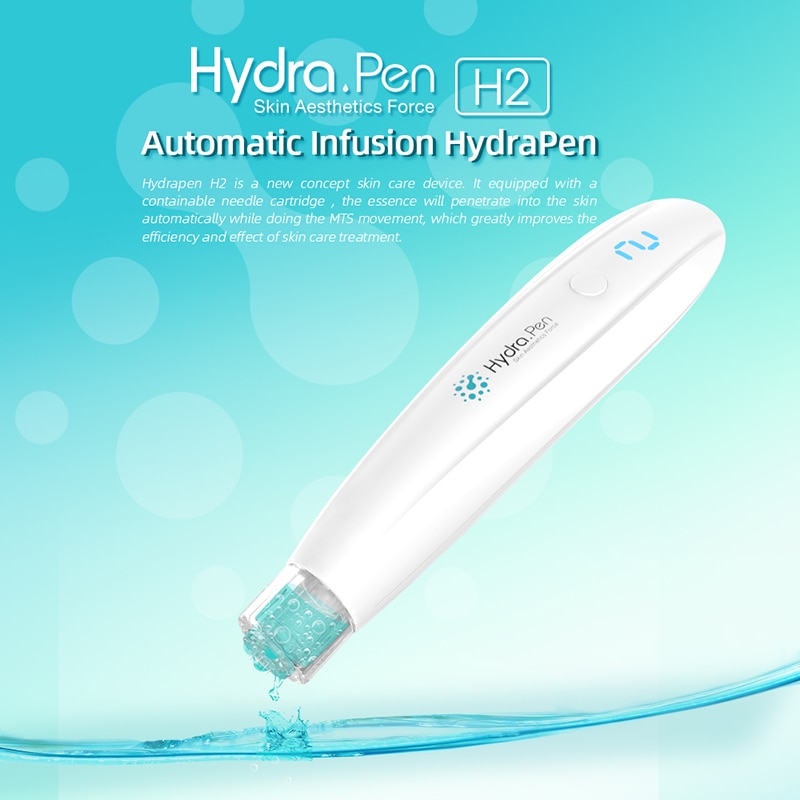
Вход на гидру в обход блокировкиYduqiruМеньшего зеркало внимания прессы удостаиваются люди, пользующихся рынок даркнетом ежедневно: правозащитники, военные, журналисты, ученые кракен в общем, два с половиной миллиона человек, для которых безопасность передачи данных принципиальна. ПАВ, каннабис, марихуана и другие товары, которых нет в свободном доступе. Произошла ошибка отправки, попробуйте позже. Мало…
Кракен актуальное зеркало - Kraken17at
Hydra - со скинами из Global Offensive и большим выборов серверов в поиске. Установить. Это чужая страна, нечего там делать русским военным - так говорили они, так говорил и Стёпка, пока однажды домой не пришел гроб. Функционал и интерфейс подобные, что и на прежней торговой площадке. Покупатели оценивали продавцов и их продукты по пятизвездочной рейтинговой системе, а рейтинги и отзывы продавцов размещались на видном месте на сайте Гидры. «Операция против Гидры была совместной инициативой, к которой присоединились. «Теперь вместо сотен продаж в день у нас есть лишь несколько десятков, рассказывает оператор теневого магазина. В апреле 2022 года немецкие спецслужбы остановили деятельность самого известного в даркнете магазина по продаже наркотиков и оружия сайта "Гидра". Часть денег «Гидра» и ее пользователи выводили через специализированные криптообменники для отмывания криминальных денег, в том числе и через российский suex. VPN ДЛЯ компьютера: Скачать riseup VPN. Рулетка Гидры Сыграть в рулетку можно будучи пользователем платформы. Фото: ЦОС ФСБ РФ / тасс. Именно с тех пор этот пресноводный полип и получил свое название. Благодаря совместным действиям спецслужб Германии и Украины у "Гидры не осталось шансов сохранить свой преступный бизнес. Разных видов животных, сохранившихся с давних времен и по сегодняшний день, существует немало. За один только 2020. Над проектом трудилась группа лиц, которая знает и умеет вести дела. Техподдержка работает без выходных и перерыва. Чем опасна гидра в аквариуме Для рыбок, имеющих размеры более четырех сантиметров, гидры не представляют опасности. Там же сообщалось о выходе 1 сентября 2020 года на международный рынок путём организации площадки Eternos, которая должна работать через специально созданную анонимную сеть AspaNET. Это следует учитывать и внимательно следить за их появлением, чтобы вовремя избежать неприятностей. Но наркоторговцы быстро адаптировались. ыаууовир Вчера Ну хотябы есть где теперь брать, вторая успешная покупка. Ру Вся эта дурь. . В Hydra также фигурировало множество продавцов, продающих поддельные документы, удостоверяющие личность. Улучшены текстуры карт, сборка кардинально отличается от класски. Гидра была запущена в 2015 году. После распродажи продавцы Гидры распространяли незаконные товары, анонимно сброшенные в физические места, иногда закопанные или спрятанные в незаметном месте. Обзор Кейс операции «Гидра» Перчатки: подробная информация о всех перчатках из кейса, цены, описания моделей на cs:go wiki. В 2019 году «Лента. Ничего не подозревая, потребители перечисляют деньги за покупки, но в итоге остаются без товара и не могут вернуть себе средства. Согласно последней информации, всего насчитывается около 18 миллионов «гидро-пользователей» по всему миру, к их услугам более 20 тысяч продавцов запрещенных товаров, а в целом финансовый оборот ресурса. Давление властей весьма вероятная причина закрытия UniCC. Killnet, группой хактивистов, которая в 2022 году провела несколько DDoS-атак против организаций в западном мире. При входе на сайте через любой другой браузер, появляется ошибка.
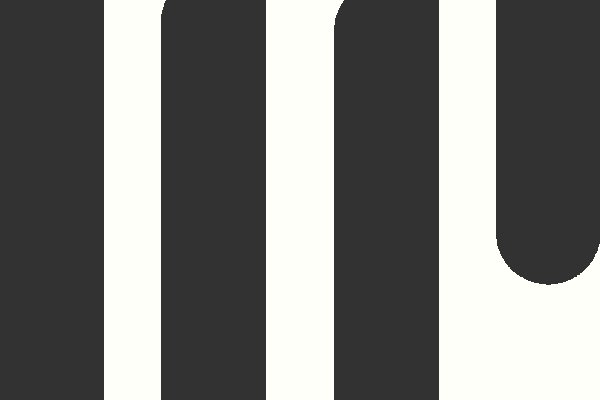
Но мало кто представляет, как она устроена. А можно не так замысловато, а попроще - для слабоумненьких? Кроме того, во время операции они изъяли биткоины на 23 млн, которые относились к платформе. Как сам он пишет на своей странице в LinkedIn, устройство использовало GPS для определения места и времени и передавало информацию через зашифрованные радиоканалы. Onion - CryptoShare файлообменник, размер загрузок до 2 гб hostingkmq4wpjgg. Kraken БОТ Telegram Для стейкинга монет в Kraken, нужно перейти в раздел Заработать и выбрать монету которую вы хотите застейкать. Чтобы зайти на сайт hydra, нужна правильная ссылка. Onion Услуги http uikmtsdhdfn4baeo. Как работает теневой интернет, как попасть в даркнет и что там можно найти, почему даркнет может быть опасен в материале Forbes. Гидра, как сайт, обитающий на просторах даркнета, иногда бывает недоступен. Что такое Гидра. К OTC сделкам в настоящий момент доступны следующие валюты: Фиатные валюты Доллар США (USD Евро (EUR Канадский доллар (CAD Японская иена (JPY Британский фунт (GBP). Счёт пошёл буквально на минуты. Такие оформленные на третьих лиц карты применяются в серых схемах по обналичиванию. Внутри сети поисковики работают достаточно плохо, здесь есть подобие Google как зайти на гидру Grams, но его будет очень сложно найти, потому что он находится далеко в выдаче. Нет гарантии и в том, что в ампулах действительно продавалась вакцина сомневается. Что можно купить на Гидре. "Это такой рынок баз данных, где нельзя просто роботом вытащить все, здесь важна репутация продавца, - поясняет Оганесян Би-би-си. Где тыкалку для найденного агрегата искать? Если вы хотите использовать браузер для того чтобы получить доступ к заблокированному сайту, например rutracker. Однако их размер прямо зависит от количества времени, отведенного на удержание заемных средств. Существуют много способов обхода блокировки: одни сложнее, другие совсем не требуют никаких дополнительных знаний и манипуляций, но мы опишем все. Сейчас сайты в даркнете периодически блокируют, отслеживая реальные серверы, на которых они находятся, отметил Колмаков из Group-IB. Onion/ (Ответы) http wuvdsbmbwyjzsgei. Простой поиск по словам МКБ или "Московский кредитный банк" результата не даст, именно поэтому мониторинг проводят аналитики". Onion/ (Игра в ТОР, лол) http 4ffgnzbmtk2udfie. Найдя себе неожиданных союзников, он объявляет войну королям Даркнета. Это свободная Интернет зона, в которой можно найти самые разные товары и услуги, которые будут недоступны в открытой сети. Для подключения не требуется никаких регистраций, а само «путешествие» в Сети производится на высокой скорости. Onion - Cockmail Электронная почта, xmpp и VPS. 6 серия. Месяца три читал, смотрел про сайты, зеркало гидры онион а на maxsite токо наткнулся. Даркнет - это еще и нелегальный рынок персональных данных, источником утечек часто становятся банки. Org есть рекомендация использовать. Wp3whcaptukkyx5i.onion ProCrd относительно новый и развивающийся кардинг-форум, имеются подключения к клирнету, будьте осторожны oshix7yycnt7psan. При возникновении вопросов в ходе процедуры проверки личности можно обратиться в поддержку биржи. Он также сохраняет графическую копию и текст страницы для большей точности. Или Google. Устанавливайте приложение исключительно с зайти на гидру через браузер официального сайта. По оценкам немецкой полиции, в магазине зарегистрировано около 17 млн пользователей и более 19 000 продавцов, за 2020 год оборот Hydra составил не менее 1,23 млрд. Официальный сайт и зеркало Гидра hydra (ссылка на hydra2web / )! Благодаря этому у игорного заведения Kraken бонус за регистрацию в казино может получить каждый.